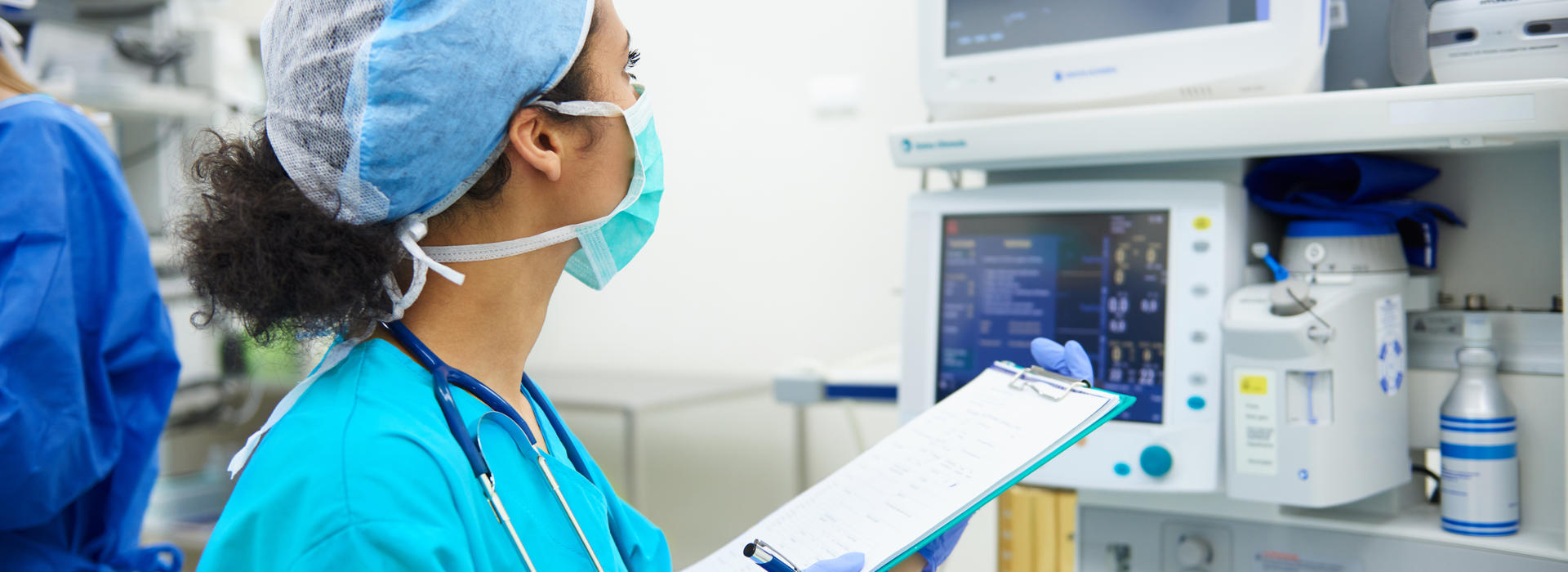
ENTRUST AI
ENTRUST AI: ENsuring the TRUSTworthiness of AI/ML Models to Optimize Continual Patient Safety
Problem and Need for the Study
Despite a rapid increase in applications of artificial intelligence (AI) and machine learning (ML) in society, the rate of adoption in medicine is lagging other areas. A critical factor to accelerate the adoption is developing trust in AI models, which hinges on reliable predictions and risk management processes. ISO 14971, Application of Risk Management to Medical Devices, is the FDA recognized consensus standard, which was originally developed for medical devices and is being used for AI/ML models. While patient populations targeted by each medical device are homogeneous, each AI/ML model can be applied to broad and varied patient populations.
Risk management for AI/ML models therefore must be individualized, targeting the individual patient rather than a population, so that patient-level differences in such varied populations can be correctly accounted for.
Innovation and Impact
In this project, we develop an individualized risk management platform and a process implementing the ISO 14971 standard for two clinical use cases, clinical deterioration, and postoperative complications, at M Health Fairview (MHFV) and Mayo Clinic.
Our first Aim is to develop computational approaches providing individualized reliability, harms, and benefits (ENTRUST AI Platform), which is a suite of models that tracks the reliability of the clinical AI predictions and predicts patient-specific harms and benefits from interventions.
Aim 2 extends current risk management best practices to the full clinical AI lifecycle building upon the ISO 14971 standard for new software devices and implementing individualized risk management processes (ENTRUST AI Process) at MHFV and Mayo. This will balance the benefits versus harms on a per-individual basis, based on information from the risk management platform.
These aims represent critical early steps towards developing a fully AI-aware clinical workflow that can help achieve the triple aims of safe, trustworthy, and fair AI in clinical practice.
Key Personnel and Performance Sites
University of Minnesota
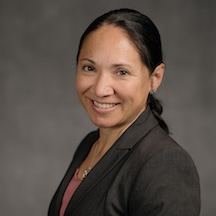
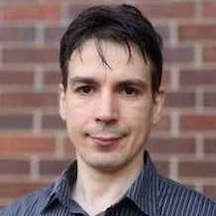
- Principal Investigators: Genevieve Melton-Meaux, Gyorgy Simon
- Co-Investigators: Christopher Tignanelli, Jenna Marquard, Vipin Kumar, Rui Zhang
- Project Manager: Molly Diethelm
Mayo Clinic
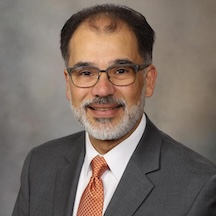
- Principal Investigators: Pedro Caraballo
- Co-Investigators: Curtis Storlie, Sean Dowdy, David Vidal
This Minnesota Partnership for Biotechnology and Medical Genomics Grant is a two-year, $1.4 million award.
Project dates: 01-July-2023 to 31-June-2025