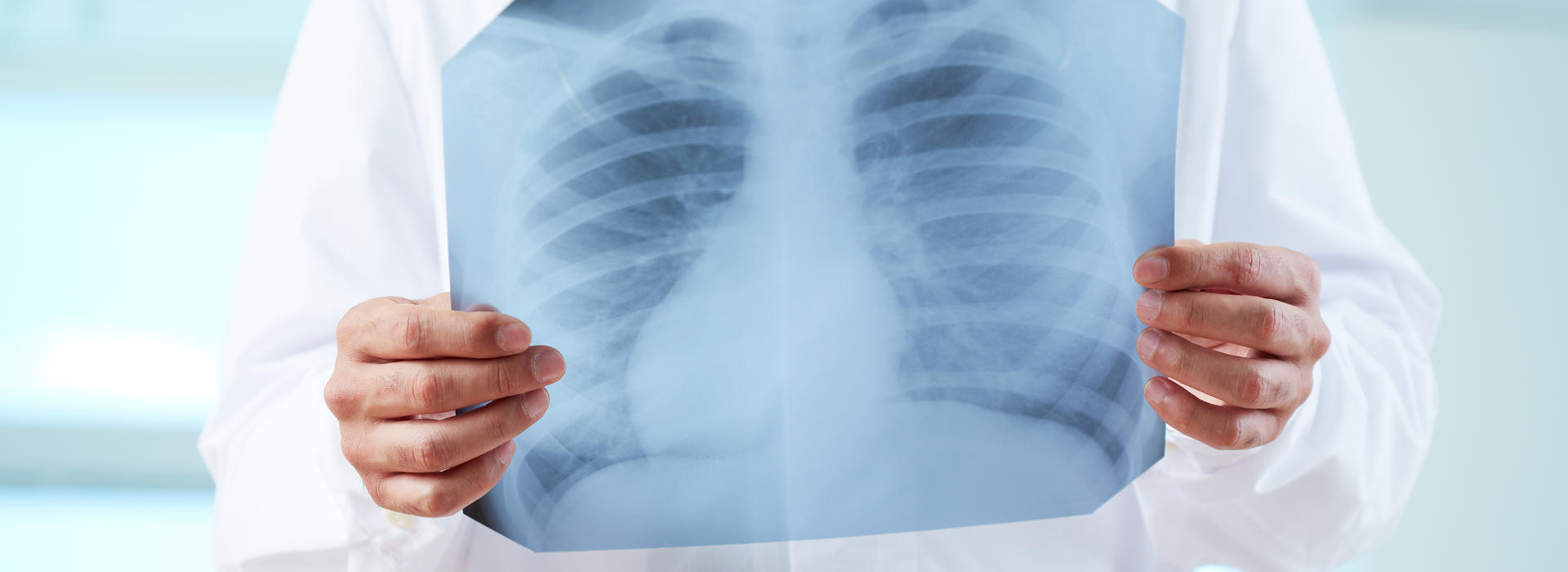
Chris Tignanelli Helps Develop Models that Predict Race from Chest X-Rays
Chris Tignanelli, MD, MS, recently collaborated with a group of researchers to determine if artificial intelligence (AI) can predict self-reported race from chest x-ray images by using pixel intensity counts.
In previous research, investigators have demonstrated that neural network models are able to predict a patient’s self-reported race from their medical images. This introduces the potential for AI models to be biased and create racial disparities.
Researchers are not sure how these models predict self-reported race as there are no known features in medical images that can be used to determine race. Dr. Tignanelli and his colleagues wanted to know if AI models are potentially using the grayscale values of image pixels to make their predictions.
To answer this question, the researchers counted the number of pixels of each grayscale value, or pixel intensity, in a large dataset of chest x-rays. They then performed a statistical test to see if different racial groups have different pixel intensity counts and trained machine learning models to predict self-reported race based on these counts.
Their statistical test shows that there is a significant difference in pixel intensity counts between different racial groups. The models they developed were also able to predict someone’s self-reported race based on their count, but they were less accurate than neural networks developed in previous studies that based their predictions on entire medical images.
Overall, the researchers were not sure if the AI models developed in previous studies used pixel intensity to predict self-reported race. However, this research demonstrates that race is embedded in medical images in ways that are not always apparent to human observers.